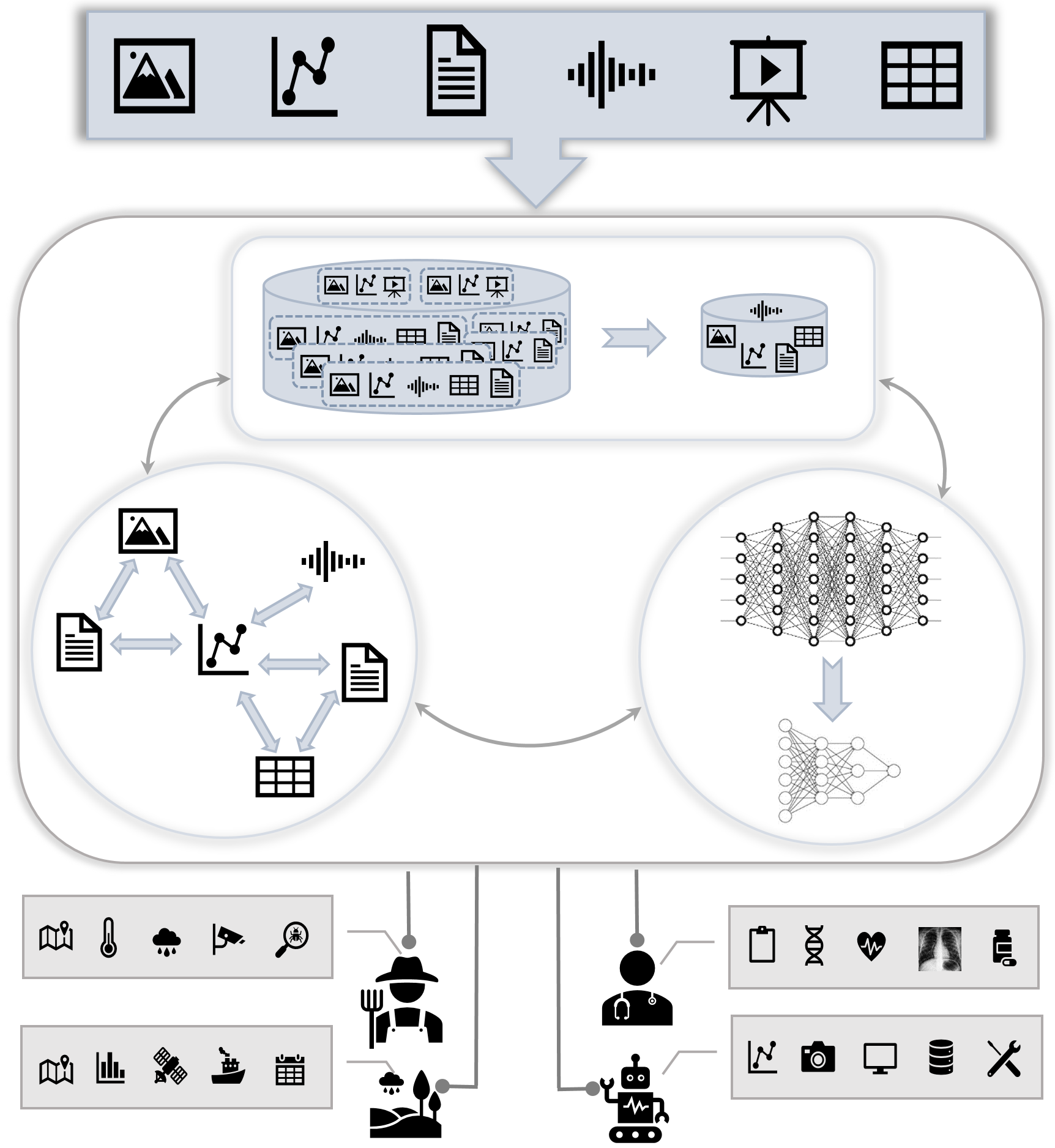
EMuLe
Enhancing Data and Model Efficiency in Multimodal Learning
Contact
Funding
BMBF
Summary
The rapid advancement of large neural networks, including Transformers and Large Language Models, has transformed the machine learning landscape. While these models exhibit extraordinary capabilities, their training demands copious amounts of data and computational resources. However, beneath the sheen of their astonishing accomplishments lies a significant challenge—data efficiency and resource optimization. This challenge becomes even more pronounced when these models are applied to multimodal data, where memory and computational efficiency are vital. Most existing studies in multimodal learning predominantly focus on image and text modalities. However, time series data, with its specific characteristics, presents another rich source of information in various applications, including sensor analysis in intelligent manufacturing, energy management, precision agriculture, and precision healthcare applications. The challenge of processing such multimodal data is magnified in scenarios where data resources are scarce, access to computational infrastructure is restricted, and cost and time factors are critical. Our goal is to propose data-efficient and model-efficient robust deep learning frameworks for multimodal data in applications with images, time series, and potentially additional modalities. Existing multimodal Transformers encounter two significant challenges in terms of efficiency: (1) data dependency and model parameter capacity: multimodal Transformers exhibit a data-hungry behavior attributed to their substantial model parameter capacity. This reliance on extensive training datasets poses a significant hurdle, limiting their applicability and scalability in real-world scenarios. Our motivation is to enhance data quality over data quantity, mitigating this data dependency and enabling these models to operate effectively even with limited training resources. (2) time and memory complexities in multimodal contexts: the inherent time and memory complexities of multimodal Transformers, growing quadratically with input sequence length due to self-attention, impose formidable constraints, particularly with jointly high-dimensional representations. We aim to comprehensively address these interdependent bottlenecks, seeking solutions that enhance the efficiency of training and inference processes, ultimately making multimodal Transformers more accessible and practical.
Hence, our central research questions revolve around optimizing data-efficient training techniques to reduce the data required to train these large networks and ensure model efficiency by significantly reducing model size without compromising performance. The primary research questions are:
• “How can we optimize data-efficient training techniques to minimize the data volume required for training Transformers and large neural networks while maintaining model performance?”
• “How can we employ model-efficient strategies to significantly reduce the model size of large neural networks while achieving comparable results?”
Duration
01.09.2024 – 31.08.2027